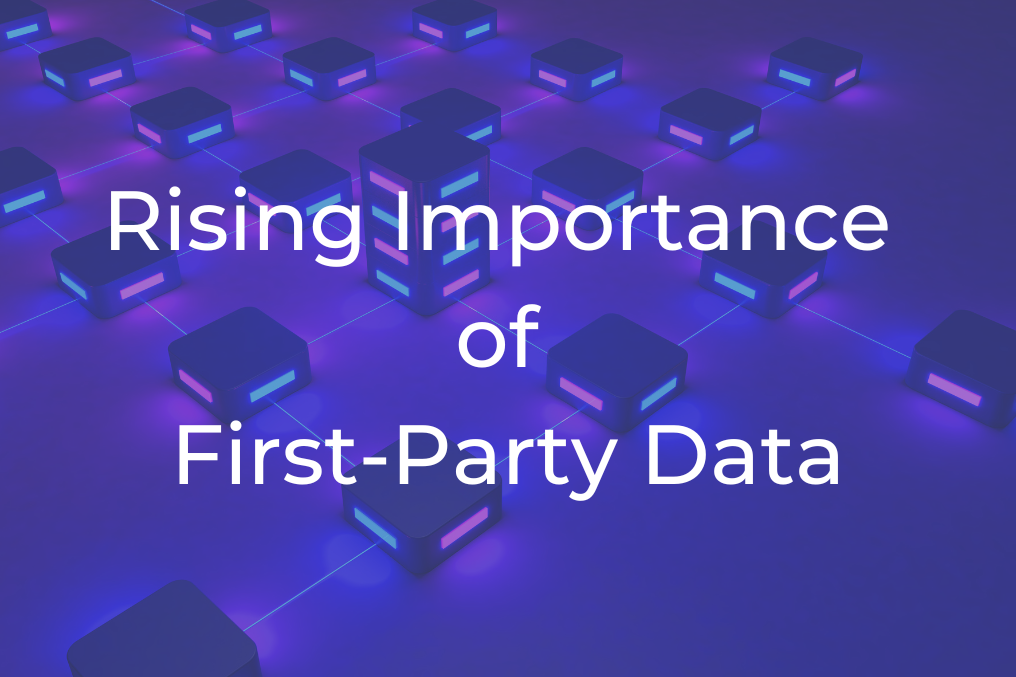
“We’ll help you boost performance, simplify processes, and drive growth with data solutions.”
In today’s data-driven world, businesses rely heavily on data warehousing solutions to store, manage, and analyze vast amounts of information. As organizations seek to leverage their data for strategic insights, choosing the right cloud data warehouse becomes critical. This blog provides a comprehensive analysis of three leading platforms: Google BigQuery, AWS Redshift, and Azure Synapse Analytics. We will explore their features, pricing models, performance, and use cases to help you make an informed decision for your data analytics needs.
Table of Contents
ToggleThe digital landscape is evolving rapidly, and with it comes the necessity for robust data warehousing solutions. Companies are inundated with data from various sources, making it imperative to have a reliable system in place to harness this information effectively. The right choice of a data warehouse can significantly impact an organization’s ability to analyze data and derive actionable insights.
In this comparative analysis, we will delve into the strengths and weaknesses of Google BigQuery, AWS Redshift, and Azure Synapse Analytics. By examining aspects such as architecture, pricing models, scalability, performance, and security features, we aim to provide a nuanced understanding of how these platforms can revolutionize your business operations.
Cloud data warehousing refers to the storage of large volumes of structured and semi-structured data in a cloud environment. Unlike traditional on-premises solutions, cloud-based warehouses offer scalability, flexibility, and cost-effectiveness. They allow businesses to store vast amounts of data without the need for extensive physical infrastructure.
Cloud data warehousing offers transformative advantages for businesses looking to optimize their data management and analytics. Here’s why it’s a game-changer:
Google BigQuery is a fully managed serverless data warehouse that enables super-fast SQL queries using the processing power of Google’s infrastructure. It is designed for large-scale analytics and offers features that simplify the management of big data.
Cloud data warehousing comes packed with features that simplify operations and drive smarter decisions. Here’s a closer look:
Amazon Redshift is a powerful cloud-based data warehouse service that allows users to run complex queries against large datasets. It utilizes columnar storage technology and massively parallel processing (MPP) to enhance query performance.
Azure Synapse Analytics is a game-changing solution that seamlessly blends big data analytics with traditional data warehousing, offering businesses a single, unified platform for managing and analyzing their data.
To facilitate a clearer understanding of these platforms, we will compare them across several critical dimensions:
Feature | Google BigQuery | AWS Redshift | Azure Synapse Analytics |
Architecture | Serverless | Cluster-based | Integrated (Big Data + Data Warehousing) |
Storage Type | Columnar | Columnar | Columnar |
Scalability | Automatic scaling | Manual scaling | Automatic scaling |
Pricing Model | Pay-per-query / Flat-rate | On-demand / Reserved Instances | Pay-per-use / Provisioned |
Performance | High performance for large queries | Optimized for complex queries | Fast processing with MPP |
Security | Google Cloud IAM | AWS IAM | Azure Active Directory |
When evaluating cloud data warehousing solutions, it’s essential to understand the key performance differences between Google BigQuery, AWS Redshift, and Azure Synapse Analytics. Each platform has unique strengths and capabilities that can significantly impact data processing and analytics performance. Here’s a detailed comparison based on various performance metrics.
When comparing the pricing models of Google BigQuery, AWS Redshift, and Azure Synapse Analytics in real-world scenarios, it’s essential to understand how each platform structures its costs based on usage, storage, and query execution. Here’s a detailed breakdown of their pricing models and how they perform under various conditions.
Data Volume | BigQuery Cost | Redshift Cost | Azure Synapse Cost |
100 GB | $0.49 | $2.24 | $1.95 |
1 TB | $5.00 | $23.00 | $20.00 |
10 TB | $50.00 | $230.00 | $200.00 |
Choosing the right cloud data warehouse—whether it be Google BigQuery, AWS Redshift, or Azure Synapse Analytics—depends largely on your organization’s specific needs regarding scalability, performance, cost structure, and existing infrastructure. Each platform offers unique advantages that can significantly enhance your analytics capabilities.
As businesses increasingly rely on data-driven decision-making, investing in a robust cloud data warehouse can pave the way for transformative insights and operational efficiency. By understanding the comparative strengths of these platforms, organizations can make informed choices that align with their strategic goals.
In conclusion, whether you are looking to optimize marketing measurement through detailed analytics or enhance product analytics capabilities within your organization, selecting the right cloud data warehouse will empower you to maximize the value of your business’s data assets.
“We’ll help you boost performance, simplify processes, and drive growth with data solutions.”
From Cookies to Customer Loyalty: The Rising Importance of First Party Data
Elevate Your Shopify Analytics: Implementing a Data Layer with GTM
GA4 and Server-Side Tagging: The Next Big Thing in Data Analytics in 2025 🚀
Cloud Data Warehouse Showdown: BigQuery, AWS Redshift, and Azure Synapse
Optimizing ETL/ELT Processes for Enhanced Data Quality: Strategies for Businesses
Unlock 2025 Marketing Potential: The Ultimate Guide to Using GA4 for Smarter Strategies
Explore related posts.
From Cookies to Customer Loyalty: The Rising Importance of First Party Data
Elevate Your Shopify Analytics: Implementing a Data Layer with GTM
GA4 and Server-Side Tagging: The Next Big Thing in Data Analytics in 2025 🚀
Cloud Data Warehouse Showdown: BigQuery, AWS Redshift, and Azure Synapse
Optimizing ETL/ELT Processes for Enhanced Data Quality: Strategies for Businesses
Subscribe to our newsletter for expert tips and actionable strategies delivered to your inbox.
Empowering businesses with data-driven insights and strategies. We specialize in analytics, marketing measurement, and optimization to fuel your growth. Let’s transform data into actionable results.